What is big data and why FinTech is buzzing about it
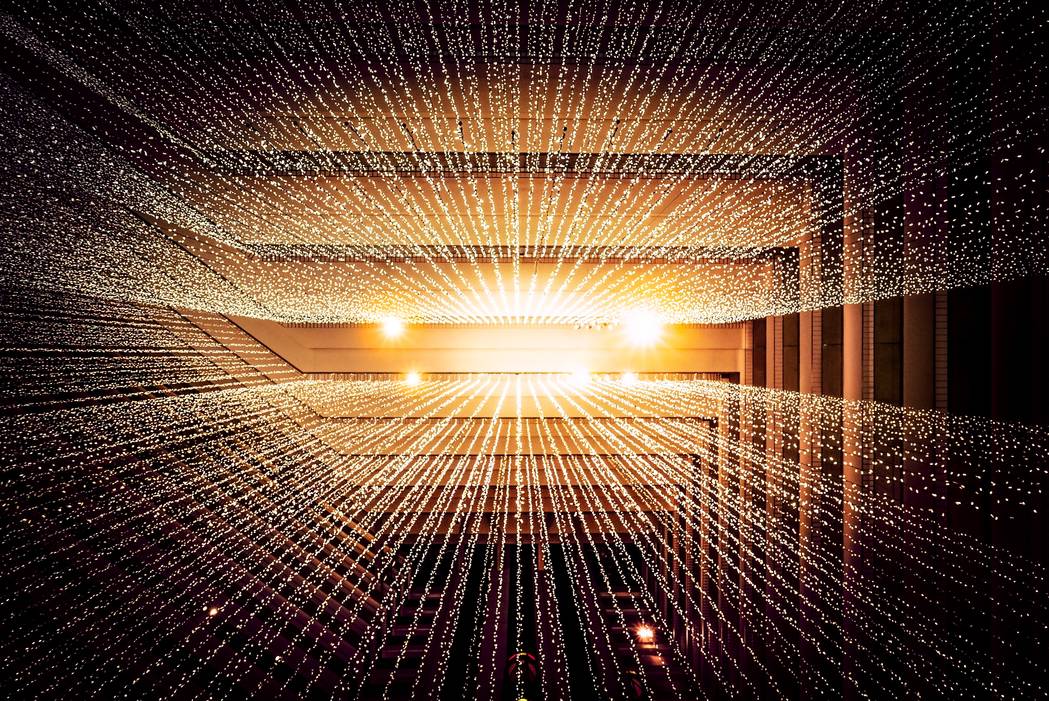
Big data is just a lot of data, isn’t it? What’s so trendy about the topic then, and why all the tittle-tattle on how complicated it is?
Well, it’s critical to grasp what kind of ‘big’ we are talking about. You might have come across 100 Gb/day figure marking the boundary. Yet, big data is not about quantity limits. It is about quantum jump. For openers, 90% of all the data in the world has been created over the last 2 years, and snowballing advance of information technologies means it’s just a beginning.
Here is some more telling numbers:
- 2.9 million emails is sent per second;
- 100 hours of video is uploaded per minute on YouTube;
- 700 billion minutes per month is spent on Facebook;
- 1.2 trillion searches per year are conducted on Google;
- 3.3 exabytes of data is sent or received by mobile devices per month.
We’ve started to generate so much more information, that no tools and methods we previously used to make sense of it can’t possibly cope with it anymore. Hence, new solutions emerge, such as MapReduce, Hardoop, NoSQL, HBase, Spark, R and many, many others.
Basically, what most of them do, is take large data bulks, split them into segments that a computer can process and analyze random segments instead of the whole bulk. As a result, analytical models are built, which are compared against other parts.
Depending on what big data tools do next, they are divided into 4 big groups:
1) Descriptive analytics simply tells what happened.
2) Diagnostic analytics goes a step further and provides a reason for why it happened.
3) Predictive analytics tries to guess what will happen next.
4) Prescriptive analytics, which is still in its infancy, suggests what you should do to get what you want.
Google, for example, can predict flu outbreaks based upon when and where people are searching for flu-related terms.
Linguists sift through Facebook statuses to learn how gender and age is affecting language use.
There are tools that parse weather data to help farmers sow the right seeds at the right time.
While big data analytics is a mother lode for researches of all kinds, in FinTech it is mostly used for fraud detection, credit scoring, and marketing.
In anti-fraud systems, big data helps to create average consumer profile, based on which fraud risk level is estimated. The more information there is on typical behavior of users, the more accurate conclusions are reached and the stronger security is maintained. Clients who leave no digital trail, for instance, are considered suspicious. Even a newly registered email may ring alarm bells.
And, of course, big data can be extremely helpful if you seek to predict customer behavior or assess borrower’s credibility. Our online purchases, photos, location signals sent by our gadgets, what we do and like in social media give away the farm. Business carefully accumulates all the info and analyzes it to offer customers what they want exactly when they want it.
In the upcoming years, the advent of the Internet of Things (IoT) will mean even more data to analyze and pinpoint each user. Also, the two main branches of Artificial Intelligence (AI), machine learning and deep learning, are expected to make a huge headway now that they are powered by big data solutions. Add here streaming analytics and edge computing growing under big data’s wing, and you, probably, won’t be surprised by IBM’s forecast that big data is going to grow into a $203 Billion dollar industry by 2020.